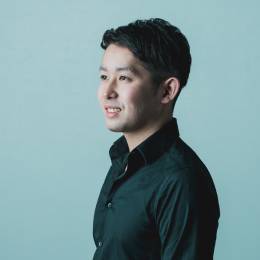
Streamlit / Dash as Frameworks for Data Apps
Streamlit and Dash are powerful frameworks that enable Python developers to build web-based data apps with ease.
Both excel at letting data scientists and analysts quickly create dashboards and reports and share them with their teams, but they clearly target different use cases:
- Streamlit: rapid data exploration for small teams
- Dash: large-scale, enterprise-ready operations
This article unpacks the strengths and feature differences behind those use cases.
The Fundamental Architectural Divide
Streamlit and Dash differ radically in the architectures they adopt. Those choices reflect each framework’s vision and become visible strengths in practice.
Streamlit | Dash | |
---|---|---|
Web server / framework | Tornado | Flask |
Strengths | Instant UI updates on user input | Horizontal server scaling |
Weaknesses | Enterprise-grade scalability, auth & authorization | Requires more front-end knowledge than pure Python |
Streamlit — A Framework for Data Scientists Building Web Apps
Streamlit was created to let data scientists turn local Python scripts into interactive web apps effortlessly. Whenever a user moves a slider or selects a filter, the script must rerun and display results in real time.
To handle those events quickly and lightly, Streamlit combines Tornado (WebSockets) with React’s virtual DOM. A single Tornado process can manage many connections, enabling lightning-fast UI updates.
This means you don’t write HTML or JavaScript callbacks—user actions are reflected instantly:
import streamlit as st
value = st.slider('Please select the value.', 0, 100, 50)
st.write(f"Selected value is {value}")
Dash — A Framework for Scalable BI Dashboards
Dash comes from the team behind Plotly. It specializes in building dashboards in Python.
Dashboards are typically team-wide tools, so enterprise scaling is crucial. Dash relies on Flask, a familiar web backend. Callbacks run per HTTP request, keeping communication stateless and avoiding in-process session storage.
As a WSGI app, Dash can run under Gunicorn with multiple workers, making horizontal scaling straightforward:
from dash import Dash, html, dcc, callback, Output, Input
import plotly.express as px
import pandas as pd
df = pd.read_csv('./data.csv')
app = Dash()
app.layout = [
html.H1('Title of Dash App', style={'textAlign':'center'}),
dcc.Dropdown(df.country.unique(), 'Canada', id='dropdown-selection'),
dcc.Graph(id='graph-content')
]
@callback(
Output('graph-content', 'figure'),
Input('dropdown-selection', 'value')
)
def update_graph(value):
dff = df[df.country == value]
return px.line(dff, x='year', y='pop')
if __name__ == '__main__':
app.run(debug=True)
Only Streamlit Stays in Pure, Simple Python
Streamlit’s WebSocket-based, real-time architecture frees you from explicit callback management.
Dash, by contrast, requires describing HTML-like components and declaring dependencies—still Python, but with front-end concepts:
app.layout = html.Div([
html.H1("My Dash App"),
dcc.Input(id='input-box'),
html.Div(id='output-div')
])
@app.callback(
Output('output-div', 'children'),
Input('input-box', 'value')
)
def update_output(value):
return f'Value: {value}'
Streamlit achieves the same with minimal code:
st.title("My Streamlit App")
value = st.text_input("Please input text")
st.write(f"Value: {value}")
Because Streamlit dedicates a Python thread to each user, you can share state easily with st.session_state
.
Enterprise Requirements Favor Dash
The Scalability Challenge
Streamlit holds a Python thread and UI objects per connection, so RAM grows linearly with users, and a load balancer must maintain session affinity—complexities when scaling out.
Dash’s proven WSGI design lets you scale infrastructure up or down with far fewer worries.
Authentication and RBAC Integration
Enterprise apps demand authentication and RBAC. Dash, especially via Dash Enterprise, integrates smoothly with Flask’s rich ecosystem—Flask-OAuthlib
, Flask-Login
, Flask-Security
, and more—so features like @login_required
are trivial to add:
from dash import Dash, html
from flask import Flask, redirect
from flask_login import LoginManager, login_user, login_required, UserMixin
server = Flask(__name__)
server.secret_key = 'your-secret-key'
login_manager = LoginManager()
login_manager.init_app(server)
class User(UserMixin):
def __init__(self, id):
self.id = id
@login_manager.user_loader
def load_user(user_id):
return User(user_id)
app = Dash(__name__, server=server)
@server.route('/login')
def login():
login_user(User('user123'))
return redirect('/')
app.layout = html.Div([
html.H1('Dash App'),
html.Div(id='content'),
])
@app.callback(
output={'id': 'content', 'property': 'children'},
inputs=[]
)
@login_required
def protected_content():
return 'You are logged in!'
if __name__ == '__main__':
app.run_server(debug=True)
Streamlit’s Tornado-plus-WebSocket model makes reusing such libraries difficult. Although st.login
and st.experimental_user
exist, you must manage auth state in st.session_state
, and sophisticated role management quickly becomes complex:
import streamlit as st
if not st.experimental_user.is_logged_in:
if st.button("Log in with Google"):
st.login()
st.stop()
if st.button("Log out"):
st.logout()
st.markdown(f"Welcome! {st.experimental_user.name}")
Conclusion
Both Streamlit and Dash let you build data apps entirely in Python, but each has distinct strengths. Many teams start with Streamlit when small, then migrate to Dash as they scale.
Our platform, Squadbase, offers scalable hosting for Streamlit. Apps deployed on Squadbase come with built-in enterprise features like authentication and access control, letting you take the codebase you began with in Streamlit and run it in production at enterprise scale.