Streamlit
Learn how to leverage Squadbase features with Streamlit.
Streamlit is an open-source library that lets you build intuitive web apps quickly with simple Python, making it perfect for internal AI applications that integrate LLM APIs to boost productivity.
With Squadbase, delivering a Streamlit app gives you all of the following out of the box:
- Deployment to an invitation-only, secure cloud environment
- Built-in user authentication
- User analytics
- Log monitoring
- In-app feedback collection from users
Sample apps
Explore apps built with Streamlit. With Squadbase, you can deploy apps like these to a secure cloud and operate them safely inside your organization.
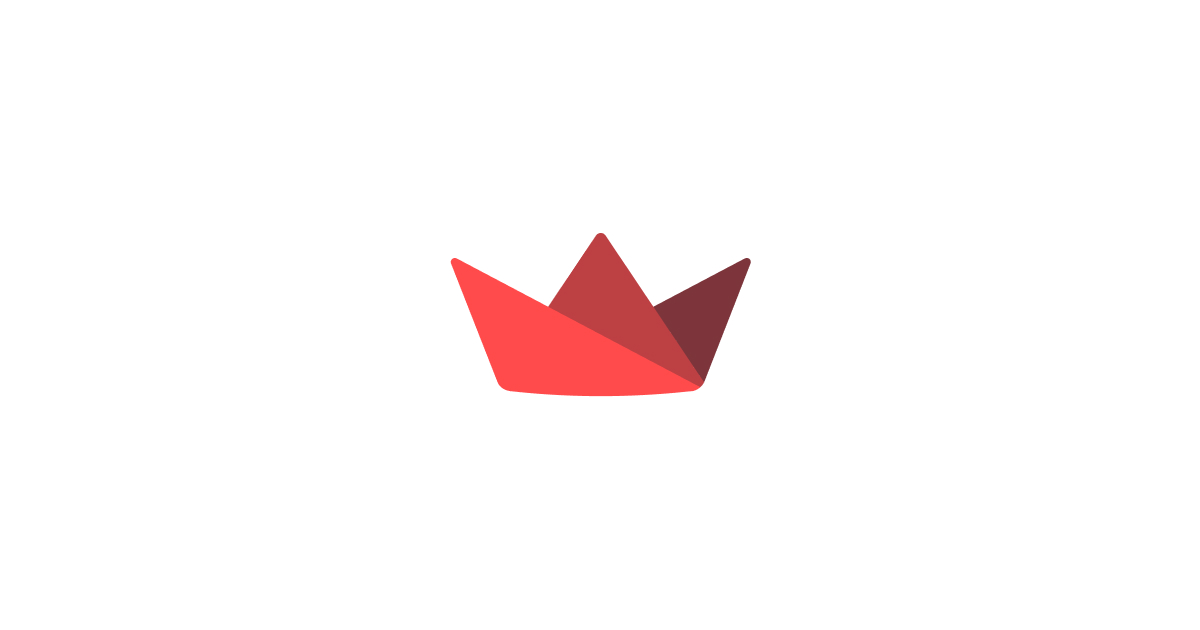
streamlit.io
App Gallery • Streamlit
Streamlit is an open-source Python framework for data scientists and AI/ML engineers to deliver interactive data apps – in only a few lines of code.
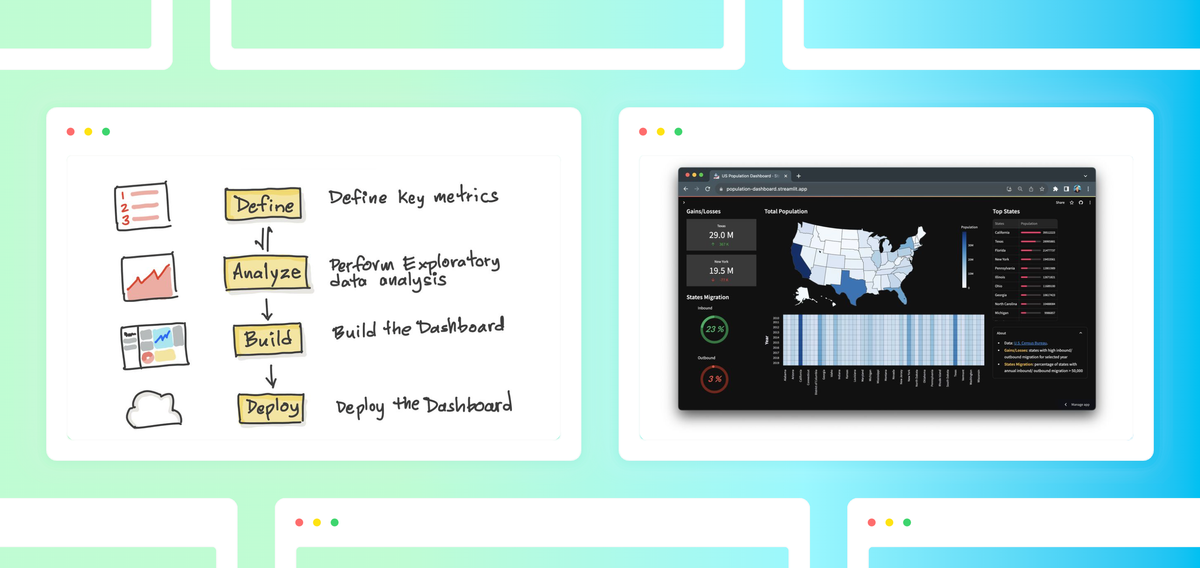
Streamlit
Building a dashboard in Python using Streamlit
Using pandas for data wrangling, Altair/Plotly for data visualization, and Streamlit as your frontend
Resources
Handy references for developing with Streamlit:
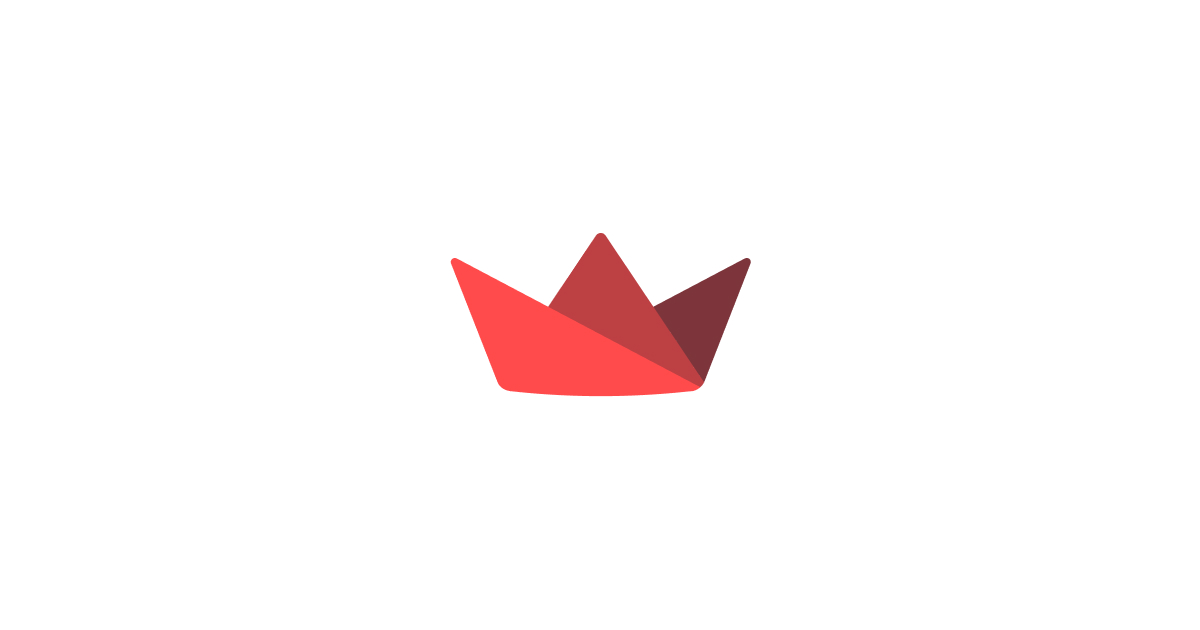
docs.streamlit.io
Official Streamlit Documentation
The official docs for Streamlit.
www.squadbase.dev
3 ways to implement user authentication with Streamlit | Squadbase Blog
Streamlit is popular for developing interactive web applications in Python for internal business applications, etc. We will compare in detail three typical methods for user authentication, which is essential for production use.
www.squadbase.dev
Streamlit vs Gradio in 2025: Comparing AI-App Frameworks | Squadbase Blog
Streamlit and Gradio are the go-to lightweight frameworks for shipping AI apps with nothing but Python in 2025. This article dissects how Streamlit’s focus on customizable UIs and operational robustness contrasts with Gradio’s strength in long-running inference and instant sharing, helping you choose the right tool for your specific use case.
www.squadbase.dev
Streamlit vs Dash in 2025: Comparing Data App Frameworks | Squadbase Blog
In 2025, Streamlit and Dash have emerged as the two most mature frameworks, each catering to a different blend of development speed and operational scale. This article reviews their underlying design principles and feature sets to help you decide which one best suits your needs.
Create a new Streamlit app
Below is the Squadbase-recommended approach using uv to set up a new project.
Initialize the project and install dependencies:
Create a starter app:
The generated file looks like this:
Run the app locally:
You’re ready to build! 🎉 For more details, see the official Streamlit docs.
Deploy with GitHub integration
Create squadbase.yml
Add squadbase.yml
to the root of your codebase—e.g. if you have app.py
and pyproject.toml
:
A minimal configuration:
See the reference for customization options.
For Streamlit, deployment.provider
currently supports only gcp
.
Import your GitHub repo into Squadbase
From the dashboard home, import your GitHub repository. Deployment starts automatically after the import completes.
Push and deploy
Commit changes, push to GitHub, and a new deployment kicks off automatically.
Make the most of Squadbase features
Squadbase offers more than deployment—it streamlines development and operations for internal apps.
User authentication & member management
When a Squadbase team member opens your app, authentication happens automatically. If the app isn’t public, only authenticated users can access it. Each user can also have a project role.
From Streamlit Python code, you can fetch user info (including project roles) and tailor behavior accordingly:
To fetch Squadbase user info from Streamlit, install squadbase-streamlit.
User analytics
Squadbase automatically collects access logs and exposes usage analytics—no extra code required.
Log monitoring
View runtime and access logs for each deployment (and each version) directly in the dashboard.
Feedback
Every deployed app includes a comment box so team members can submit feedback.