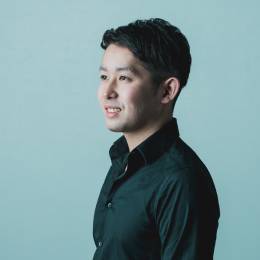
Streamlit / Gradio as Frameworks for AI Apps
Streamlit and Gradio are both popular for letting you build interactive web apps using nothing but Python.
Although they share the “light-weight Python code → web app” philosophy, their focus differs sharply:
- Streamlit = strong at customization and complex UI/UX
- Gradio = strong at publishing ML models and running long-lasting inference jobs
Below we examine each framework’s strengths and use-cases when you’re building and operating AI apps inside an organization.
Streamlit Use-Cases — Combining “Presentation” and “Operational Robustness”
Streamlit shines where you need the ease of building interactive web apps and the flexibility to handle feature requests that crop up once the tool is in production.
UI Customization
In addition to built-in widgets like st.line_chart
and st.button
, there’s a rich ecosystem of community components such as streamlit-extras and st-chat.
Because you can inject CSS or leverage the theme API, you can freely match corporate brand colors and layouts.
import streamlit as st
# Create a button
if st.button('Click me'):
st.write('Button clicked!')
# Customize layout and theme using CSS
st.markdown(
"""
<style>
.stButton>button {
background-color: #4CAF50;
color: white;
}
</style>
""",
unsafe_allow_html=True
)
Hybrid Data + AI Apps
By layering embeddings or LLM outputs on top of st.dataframe
or st.altair_chart
, you can naturally implement compound UIs such as “a chat app with charts.”
Streamlit originally targeted data scientists, so it supports many visualization libraries and excels at presenting data.
Components for real-time AI interaction—for example st.chat_message
for streaming chat updates—have also matured, making it easy to build hybrid apps that mix data and AI.
import streamlit as st
import pandas as pd
import altair as alt
st.set_page_config(layout="wide")
col1, col2 = st.columns([1, 1])
data = pd.DataFrame({
'Category': ['A', 'B', 'C'],
'Value': [10, 20, 30],
'LLM Inference': ['Result A', 'Result B', 'Result C']
})
with col1:
# Display the data
st.dataframe(data, use_container_width=True)
# Bar chart
chart = alt.Chart(data).mark_bar(color='#4A90E2').encode(
x=alt.X('Category', axis=alt.Axis(labelAngle=0)),
y=alt.Y('Value', title='Value'),
tooltip=['Category', 'Value', 'LLM Inference'],
color=alt.Color('Category', legend=None)
).properties(height=300)
st.altair_chart(chart, use_container_width=True)
with col2:
st.title("Query About Data")
if "messages" not in st.session_state:
st.session_state.messages = []
for m in st.session_state.messages:
with st.chat_message(m["role"]):
st.markdown(m["content"])
prompt = st.chat_input("Enter your question")
if prompt:
with st.chat_message("user"):
st.markdown(prompt)
st.session_state.messages.append({"role": "user", "content": prompt})
with st.spinner("Getting response from OpenAI…"):
# call OpenAI API
with st.chat_message("assistant"):
answer = "This sample dataset has three categories (A, B, C) with values 10, 20, 30."
st.markdown(answer)
st.session_state.messages.append({"role": "assistant", "content": answer})
Reliability in Production
Because Streamlit runs each user session as an isolated script, you don’t worry about global-state clashes and side effects, which lowers debugging overhead.
Its weakness is managing very long-running ML inference or high concurrent loads; you typically off-load heavy jobs to a separate API (FastAPI, etc.) and have Streamlit poll or use WebSockets for progress updates.
Use-Cases Best Suited to Streamlit
- Internal dashboards + AI assistants
- Operational tools with complex forms or dynamic reporting
- Proof-of-concepts that should move straight to branded production
Gradio Use-Cases — Strong at Publishing AI/ML Models & Long-Running Inference
Gradio offers less UI flexibility than Streamlit but focuses on making ML models easy to share. Its simple, highly intuitive API ships only the components you really need.
Key advantages include ready-made widgets for tasks Streamlit lacks out of the box (image generation, speech recognition, etc.) and built-in helpers that simplify inference job management.
Components Specialized for Model I/O
Widgets like gr.Audio
, gr.Image
, and video streams turn multimedia I/O into one-liners—perfect for showing trained models.
Even a voice-recording app that transcribes audio takes only a few lines:
import gradio as gr
def transcribe_audio(audio):
return "Audio has been recorded. In a real application, speech recognition would be performed here."
demo = gr.Interface(
fn=transcribe_audio,
inputs=gr.Audio(type="filepath"),
outputs="text",
title="Speech Recognition Demo",
description="Record audio with your microphone and convert it to text."
)
if __name__ == "__main__":
demo.launch()
gr.Flagging
automatically stores user feedback under .gradio/flagged
, giving you a zero-code feedback loop for continual learning.
Smart Execution of “Long Inference” with Queues
Gradio ships with an async backend queue that scales to thousands of concurrent users. Components like progress bars keep UX smooth even for tasks that take tens of seconds or minutes (image generation, speech-to-speech, etc.).
The sample below caps concurrency at 2 (ideal when you have only two GPUs) and uses gr.Progress
to show real-time status:
import gradio as gr
import time
def image_gen(prompt, progress=gr.Progress()):
progress(0, desc="Starting")
time.sleep(1)
progress(0.5)
time.sleep(1)
progress(1)
return "https://www.gradio.app/_app/immutable/assets/gradio.CHB5adID.svg"
with gr.Blocks() as demo:
prompt = gr.Textbox()
image = gr.Image()
btn1 = gr.Button("Generate Image via model 1")
btn2 = gr.Button("Generate Image via model 2")
btn3 = gr.Button("Generate Image via model 3")
btn1.click(image_gen, prompt, image, concurrency_limit=2, concurrency_id="gpu_queue")
btn2.click(image_gen, prompt, image, concurrency_id="gpu_queue")
btn3.click(image_gen, prompt, image, concurrency_id="gpu_queue")
demo.launch()
Instant Sharing & Instant API Generation
Set demo.launch(share=True)
and your Gradio app is hosted on Hugging Face with one line. No cloud setup—just tweak your local code and share the link.
Hosting on Hugging Face Spaces or your own server also auto-generates REST/WS APIs (curl, Python, JavaScript) whose I/O mirrors the demo UI.
While the UI is less customizable, the framework automates every step of demo distribution.
Note, though, that public share links expire in a week and rely on Gradio/Hugging Face infra, so long-term internal apps with sensitive data require extra authentication and access-control work.
Use-Cases Best Suited to Gradio
- Rapid publication of research results or model demos
- AI apps for image generation / speech recognition / TTS or other long-running inference
Conclusion
Beyond their UI components, Streamlit and Gradio diverge in how they distribute AI apps within teams. Understanding each framework’s strengths and mapping them to your use-case is critical.
Both began as tools to let you try AI/ML models in a browser, but team-level adoption demands clear thinking about execution models and operations.
When to choose which?
- Streamlit – Building complex, internal, production-grade AI apps
- Gradio – Building interactive AI demo apps that wrap model inference